The block AAA algorithm
Ion Victor Gosea and Stefan Güttel, July 2020Download PDF or m-file
Contents
Introduction
Since version 2.9 the RKToolbox provides two new utility functions util_block_aaa and util_rmse for working with rational functions represented in a generalized barycentric form with matrix-valued weights,
In this notation, the are
nonsingular weight matrices, the
are pairwise distinct barycentric support points in the complex plane, and the
are
matrices. Different from the representation of rational functions computed by the RKFIT method [1], the AAA method [5] and its surrogate [2] and set-valued variants [4], the rational function
has a nonscalar "denominator matrix polynomial." See [3] for more details and a number of numerical experiments with different representations of rational matrix-valued functions.
Demonstration
We focus on a simple matrix-valued function
to demonstrate the use of util_block_aaa. This function is defined as below and we sample it at 20 equidistant points on the interval
:
F = @(z) [ 1/(z+1) 1/(z^2-5) z ; 1/(z^2+5+1i) (2+z^2)/(z^3+3*z^2+1) 7 ]; pts = linspace(0,10,20);
Together with an error tolerance tol and a number of maximal iterations maxit, we have all that is needed to reapproximate using util_block_aaa:
opts.tol = 1e-12; opts.maxit = 10; [R,rmse,out] = util_block_aaa(F,pts,opts);
The output R is a baryfun object and we can display its info as follows:
disp(R)
BARYFUN object of block size 2-by-3 and degree 5.
The output rmse stores the root mean squared error over all sampling points for each iteration of the block AAA method. In this case, we have resolved to about machine precision after 6 iterations:
semilogy(rmse) axis tight, hold on legend('block AAA') xlabel('iteration'), ylabel('RMSE') disp(['The final RMSE is ' num2str(util_rmse(pts,F,R)) ])
The final RMSE is 2.3012e-15
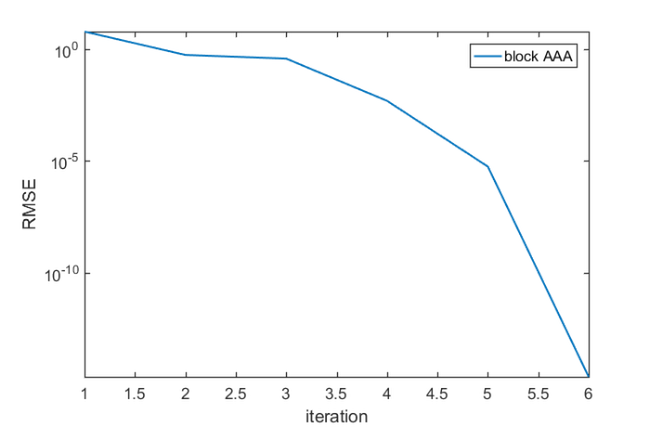
References
[1] M. Berljafa and S. Güttel. The RKFIT algorithm for nonlinear rational approximation, SIAM J. Sci. Comput., 39(5):A2049--A2071, 2017.
[2] S. Elsworth and S. Güttel. Conversions between barycentric, RKFUN, and Newton representations of rational interpolants, Linear Algebra Appl., 576:246--257, 2019.
[3] I. V. Gosea and S. Güttel. Algorithms for the rational approximation of matrix-valued functions, arXiv preprint 2003.06410v1, 2020. (https://arxiv.org/abs/2003.06410)
[4] P. Lietaert, J. Perez, B. Vandereycken, and K. Meerbergen. Automatic rational approximation and linearization of nonlinear eigenvalue problems, arXiv preprint 1801.08622, 2018. (https://arxiv.org/pdf/1801.08622.pdf)
[5] Y. Nakatsukasa, O. Sete, and L. N. Trefethen. The AAA algorithm for rational approximation, SIAM J. Sci. Comput., 40(3):A1494--A1522, 2018.